Adaptive Robotic Training for Subtractive Manufacturing
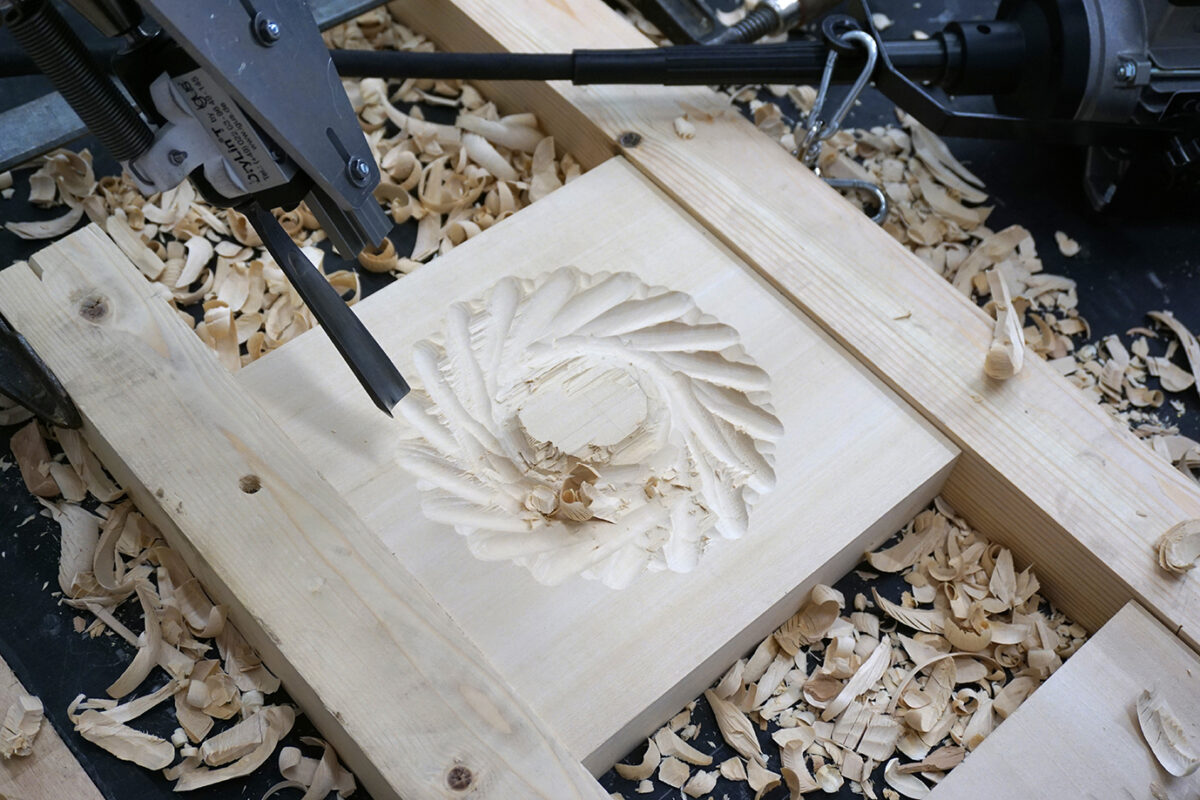
Integrating sensing with robotic fabrication procedures, the research wants to investigate potential methods for analysing qualitative material feedback and integrate them into the design environment as the fabrication unfolds. To achieve this, the research proposes the development of an adaptive framework for subtractive fabrication strategies where the stages of design and fabrication are not arranged in a linear fashion but rather in a continuously updating loop informed by sensor data and sequential training sessions.
This is articulated around two main areas: 1) An “active” simulation environment able to locally described the workpiece and store continuously updating layers of “affordances” 2) A trained robotic agent able to communicate through feedback loops and operate, increasingly well, within this environment.
The training of the system is structured, on one hand, through the recording of skilled human experts performing subtractive operations (e.g. carving procedures with a set of chisels and gouges), while on the other through “extensive” robotic explorations directly operating with the material. In both cases, the recorded information is subsequently compiled into large datasets and used within a machine learning procedure (Artificial Neural Network) to train an adaptive robotic system for subtractive fabrication processes with a similar range of materials and tools.